Quick Overview Contents
In SPSS, SELECT IF permanently removes
a selection of cases (rows) from your data.
- Example 1 - Selection for 1 Variable
- Example 2 - Selection for 2 Variables
- Example 3 - Selection for (Non) Missing Values
- Tip 1 - Inspect Selection Before Deletion
- Tip 2 - Use TEMPORARY
Summary
SELECT IF in SPSS basically means “delete all cases that don't satisfy one or more conditions”. Like so, select if(gender = 'female'). permanently deletes all cases whose gender is not female. Let's now walk through some real world examples using bank_clean.sav, partly shown below.
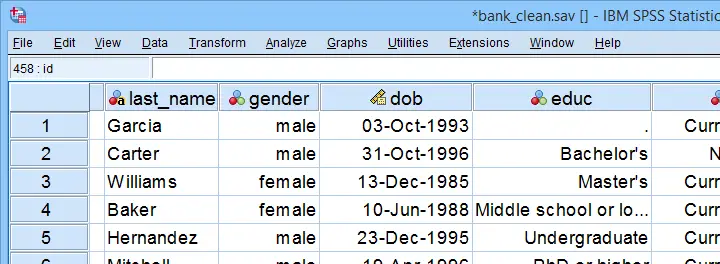
Example 1 - Selection for 1 Variable
Let's first delete all cases who don't have at least a Bachelor's degree. The syntax below:
- inspects the frequency distribution for education level;
- deletes unneeded cases;
- inspects the results.
set tnumbers both.
*Run minimal frequencies table.
frequencies educ.
*Select cases with a Bachelor's degree or higher. Delete all other cases.
select if(educ >= 4).
*Reinspect frequencies.
frequencies educ.
Result
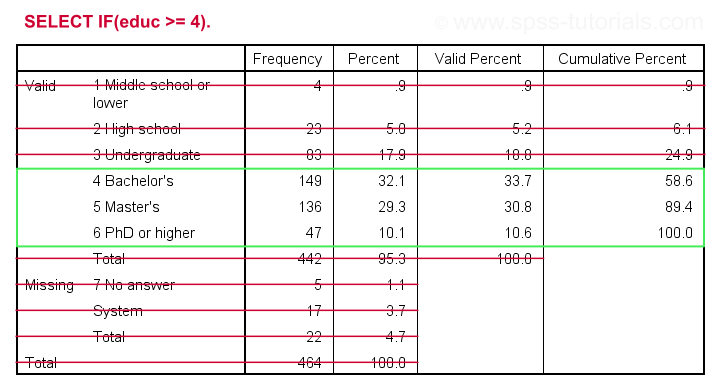
As we see, our data now only contain cases having a Bachelor's, Master's or PhD degree. Importantly, cases having
on education level have been removed from the data as well.
Example 2 - Selection for 2 Variables
The syntax below selects cases based on gender and education level: we'll keep only female respondents having at least a Bachelor's degree in our data.
crosstabs educ by gender.
*Select females having a Bachelor's degree or higher.
select if(gender = 0 & educ >= 4).
*Reinspect contingency table.
crosstabs educ by gender.
Result
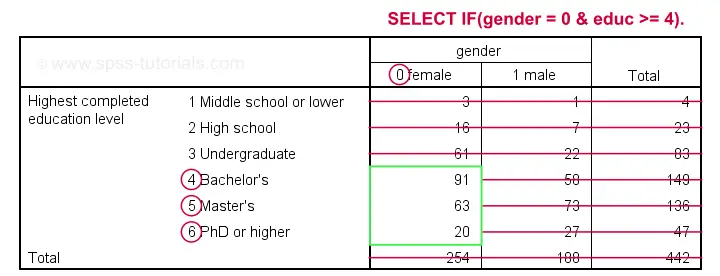
Example 3 - Selection for (Non) Missing Values
Selections based on (non) missing values are straightforward if you master SPSS Missing Values Functions. For example, the syntax below shows 2 options for deleting cases having fewer than 7 valid values on the last 10 variables (overall to q9).
select if(nvalid(overall to q9) >= 7)./*At least 7 valid values or at most 3 missings.
execute.
*Alternative way, exact same result.
select if(nmiss(overall to q9) < 4)./*Fewer than 4 missings or more than 6 valid values.
execute.
Tip 1 - Inspect Selection Before Deletion
Before deleting cases, I sometimes want to have a quick look at them. A good way for doing so is creating a FILTER variable. The syntax below shows the right way for doing so.
compute filt_1 = 0.
*Set filter variable to 1 for cases we want to keep in data.
if(nvalid(overall to q9) >= 7) filt_1 = 1.
*Move unselected cases to bottom of dataset.
sort cases by filt_1 (d).
*Scroll to bottom of dataset now. Note that cases 459 - 464 will be deleted because they have 0 on filt_1.
*If selection as desired, delete other cases.
select if(filt_1).
execute.
Quick note: select if(filt_1). is a shorthand for select if(filt_1 <> 0). and deletes cases having either a zero or a missing value on filt_1.
Result
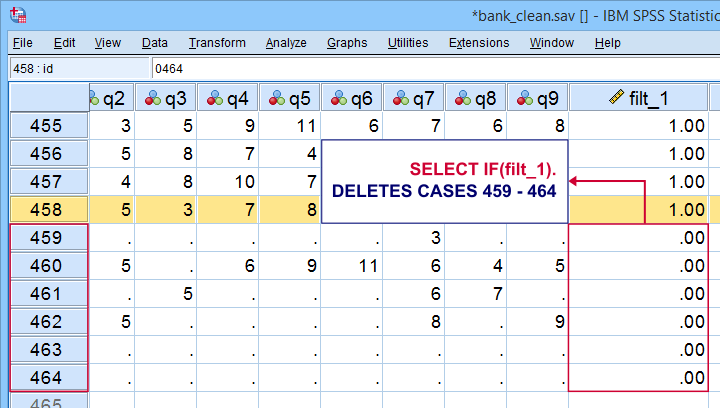
Tip 2 - Use TEMPORARY
A final tip I want to mention is combining SELECT IF with TEMPORARY. By doing so, SELECT IF only applies to the first procedure that follows it. For a quick example, compare the results of the first and second FREQUENCIES commands below.
temporary.
*Select only female cases.
select if(gender = 0).
*Any procedure now uses only female cases. This also reverses case selection.
frequencies gender educ.
*Rerunning frequencies now uses all cases in data again.
frequencies gender educ.
Final Notes
First off, parentheses around conditions in syntax are not required. Therefore, select if(gender = 0). can also be written as select if gender = 0. I used to think that shorter syntax is always better but I changed my mind over the years. Readability and clear structure are important too. I therefore use (and recommend) parentheses around conditions. This also goes for IF and DO IF.
Right, I guess that should do. Did I miss anything? Please let me know by throwing a comment below.
Thanks for reading!
THIS TUTORIAL HAS 29 COMMENTS:
By Ruben Geert van den Berg on June 19th, 2020
Hi Esmee!
This would happen if you accidentally specified 9 as missing as well. Otherwise, the syntax should do as you intended. One solution is to switch off all missing values as in
MISSING VALUES somvariable ().
before you run the SELECT IF.
P.s. I think your coding sucks. Having 9 as valid and 8 as missing is confusing. We recommend using huge (positive) values such as 999999999 and 999999998 for user missing values. Like so, it's immediately clear that these can't be valid.
Hope that helps!
SPSS tutorials
By Esmee on June 20th, 2020
Hi Ruben,
Thanks! 9 is a missing value as well, so I figured it would have had something to do with that. In the end I want to remove those cases with value 9 aswell, but I wanted to do it step by step, to see the difference between the cases (reason for missing if different for instance). I'll just figure out another way to do that then.
Thanks!
P.S. I use a dataset form an international study, so the (original)coding isn't mine. I did think it was weird though how they coded some missing values 8 and 9, and others 999999 etc indeed.
By Ruben Geert van den Berg on June 21st, 2020
I thought 9 was valid and 8 missing. That would be weird!
Using huge numbers for missings has 2 great advantages:
-it's immediately clear these are not normal values and
-if you forget to specify them as missing, some output may look absolutely crazy so you'll understand something went wrong.
If you'd forget to set 8 or 9 as missing, your output probably looks very reasonable so it doesn't draw your attention to the problem. Just my opinion...
Good luck with your project!
P.s. if you or any fellow students need any personal assistance, we offer just that via Sigma Plus Statistiek (in Dutch or English).
By Jon Peck on January 6th, 2021
A useful tool to use with SELECT CASES (and FILTER) is DATASET COPY. then you can experiment without messing up the input data.
By Ruben Geert van den Berg on January 7th, 2021
Hi Jon!
This may surprise you, but I stopped working on multiple datasets simultaneously some years ago.
I felt the DATASET ACTIVATE / DATASET NAME / DATASET CLOSE commands cluttered up my syntax too much and cost me more time than they saved me.
Also, I want projects to have as few data files as possible. The amount of work increases somewhat exponentially with the complexity of projects.
My most recent approach is to sometimes make "safety clones" of variables within a dataset and then compare these to the adjusted variables with CROSSTABS / MEANS.
I had a nice, simple tool for this (basically just running RECODE / APPLY DICTIONARY / STRING commands) but it's becoming obsolete because I haven't moved on to Python3.x yet...