SPSS ANOVA without Raw Data
- 1. Set Up Matrix Data File
- 2. SPSS Oneway Dialogs
- 3. Adjusting the Syntax
- 4. Interpreting the Output
In SPSS, you can fairly easily run an ANOVA or t-test without having any raw data. All you need for doing so are
- the sample sizes,
- the means and
- the standard deviations
of the dependent variable(s) for the groups you want to compare. This tutorial walks you through analyzing the journal table shown below.
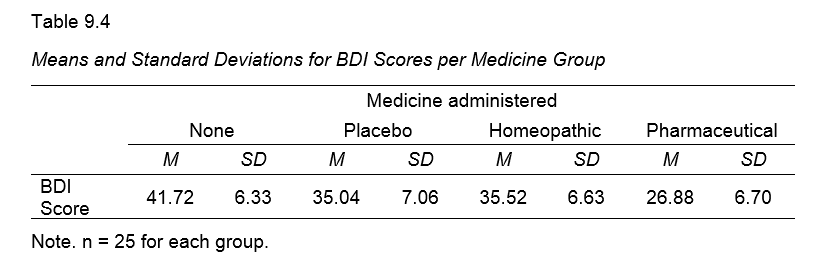
1. Set Up Matrix Data File
First off, we create an SPSS data file containing 3 rows for each group. You may use descriptives-for-anova-01.sav -partly shown below- as a starting point.
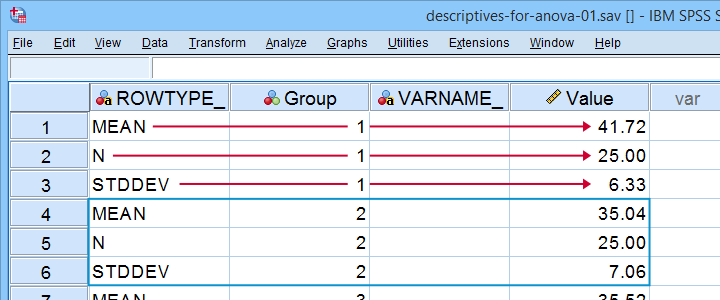
There's 3 adjustments you'll typically want to apply to this example data file:
- removing or adding sets of 3 rows if you want to compare fewer or more groups;
- changing sample sizes, means and standard deviations in the “Value” variable;
- changing the variable labels for the independent and dependent variables as indicated below.
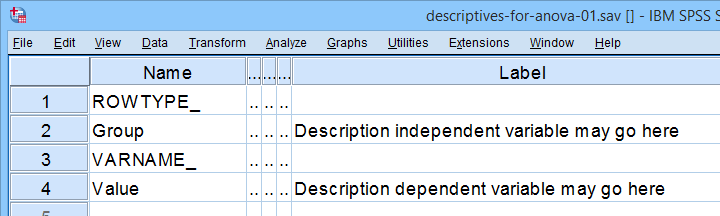
I recommend you don't make any other changes to this data file or otherwise the final analysis is likely to crash. For instance,
- don't change any variable names;
- don't change the variable order;
- don't remove the empty string variable VARNAME_
2. SPSS Oneway Dialogs
First off, make sure the example data file is the only open data file in SPSS. Next, navigate to and fill out the dialogs as if you're analyzing a “normal” data file.
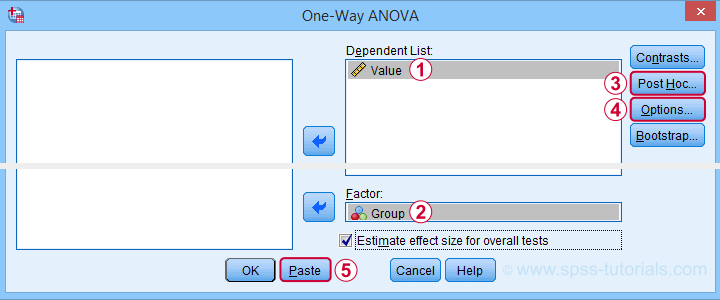
You may select all options in this dialog. However, Levene's test -denoted as Homogeneity of variances test- will not run as it requires raw data.
This is no problem for the data at hand due to their equal sample sizes. For other data, it may be wise to carefully inspect the Welch test as discussed in SPSS ANOVA - Levene’s Test “Significant”.
Anyway, completing these steps results in the syntax below. But don't run it just yet.
ONEWAY Value BY Group
/ES=OVERALL
/STATISTICS DESCRIPTIVES WELCH
/PLOT MEANS
/MISSING ANALYSIS
/CRITERIA=CILEVEL(0.95)
/POSTHOC=TUKEY ALPHA(0.05).
3. Adjusting the Syntax
Note that we created syntax just like we'd do when analyzing raw data. You could run it, but SPSS would misinterpret the data as 4 groups of 3 observations each. For SPSS to interpret our matrix data correclty, add /MATRIX IN(*). as shown below.
ONEWAY Value BY Group
/ES=OVERALL
/STATISTICS DESCRIPTIVES WELCH
/PLOT MEANS
/CRITERIA=CILEVEL(0.95)
/POSTHOC=TUKEY ALPHA(0.05)
/matrix in(*).
*CORRECTED SYNTAX FOR SPSS 26 OR LOWER.
ONEWAY Value BY Group
/STATISTICS DESCRIPTIVES WELCH
/PLOT MEANS
/CRITERIA=CILEVEL(0.95)
/POSTHOC=TUKEY ALPHA(0.05)
/matrix in(*).
4. Interpreting the Output
First off, note that SPSS has understood that our data file has 4 groups of 25 observations each as shown below.
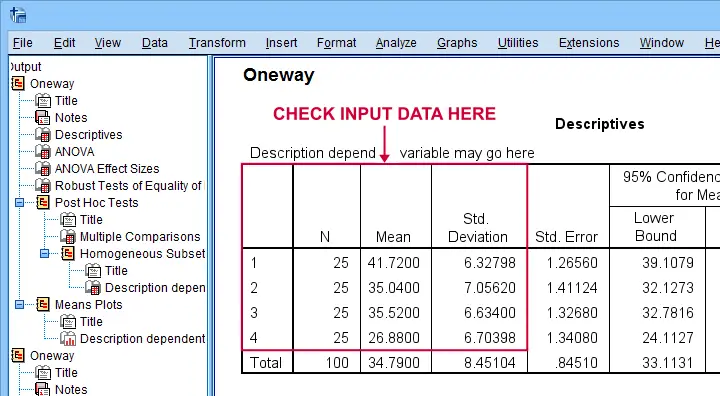
The remainder of the output is nicely detailed and includes
- the main ANOVA F-test;
- post-hoc tests (Tukey's HSD);
- the Welch test (not really needed for this example);
- a line chart visualizing our sample means;
- various effect size measures such as partial eta squared (only SPSS version 27+).
The main output you cannot obtain from these data are
- Levene's test for the homogeneity assumption;
- the Kolmogorov-Smirnov normality test and;
- the Shapiro-Wilk normality test.
For the sample sizes at hand, however, none of these are very useful anyway. A more thorough interpretation of the output for this analysis is presented in SPSS - One Way ANOVA with Post Hoc Tests Example.
Right, so I hope you found this tutorial helpful. We always appreciate if you throw us a quick comment below. Other that that:
Thanks for reading!
SPSS ANOVA with Post Hoc Tests
Contents
- Descriptive Statistics for Subgroups
- ANOVA - Flowchart
- SPSS ANOVA Dialogs
- SPSS ANOVA Output
- SPSS ANOVA - Post Hoc Tests Output
- APA Style Reporting Post Hoc Tests
Post hoc tests in ANOVA test if the difference between
each possible pair of means is statistically significant.
This tutorial walks you through running and understanding post hoc tests using depression.sav, partly shown below.
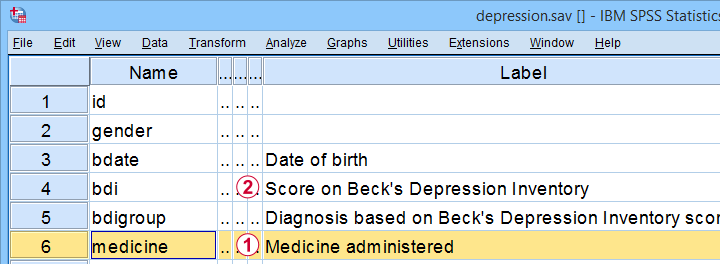
The variables we'll use are
the medicine that our participants were randomly assigned to and
their levels of depressiveness measured 16 weeks after starting medication.
Our research question is whether some medicines result in lower depression scores than others. A better analysis here would have been ANCOVA but -sadly- no depression pretest was administered.
Quick Data Check
Before jumping blindly into any analyses, let's first see if our data look plausible in the first place. A good first step is inspecting a histogram which I'll run from the SPSS syntax below.
frequencies bdi
/format notable
/histogram.
Result
First off, our histogram (shown below) doesn't show anything surprising or alarming.
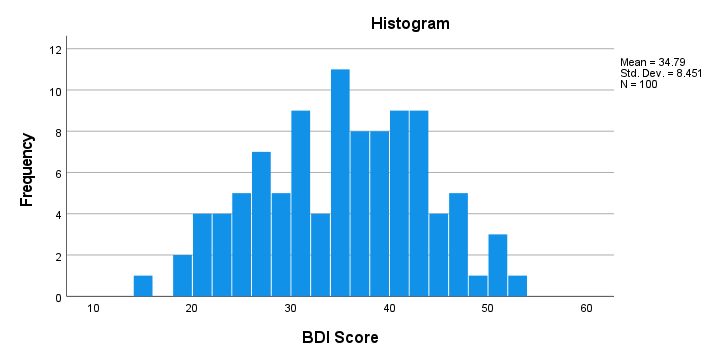
Also, note that N = 100 so this variable does not have any missing values. Finally, it could be argued that a single participant near 15 points could be an outlier. It doesn't look too bad so we'll just leave it for now.
Descriptive Statistics for Subgroups
Let's now run some descriptive statistics for each medicine group separately. The right way for doing so is from
or simply typing the 2 lines of syntax shown below.
means bdi by medicine
/cells count min max mean median stddev skew kurt.
Result
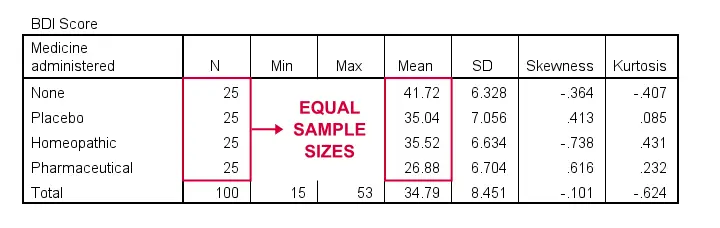
As shown, I like to present a nicely detailed table including the
for each group separately. But most important here are the sample sizes because these affect which assumptions we'll need for our ANOVA.
Also note that the mean depression scores are quite different across medicines. However, these are based on rather small samples. So the big question is: what can we conclude about the entire populations? That is: all people who'll take these medicines?
ANOVA - Null Hypothesis
In short, our ANOVA tries to demonstrate that some medicines work better than others by nullifying the opposite claim. This null hypothesis states that
the population mean depression scores are equal
across all medicines.
An ANOVA will tell us if this is credible, given the sample data we're analyzing. However, these data must meet a couple of assumptions in order to trust the ANOVA results.
ANOVA - Assumptions
ANOVA requires the following assumptions:
- independent observations;
- normality: the dependent variable must be normally distributed within each subpopulation we're comparing. However, normality is not needed if each n > 25 or so.
- homogeneity: the variance of the dependent variable must be equal across all subpopulations we're comparing. However, homogeneity is not needed if all sample sizes are roughly equal.
Now, homogeneity is only required for sharply unequal sample sizes. In this case, Levene's test can be used to examine if homogeneity is met. What to do if it isn't, is covered in SPSS ANOVA - Levene’s Test “Significant”.
ANOVA - Flowchart
The flowchart below summarizes when/how to check the ANOVA assumptions and what to do if they're violated.
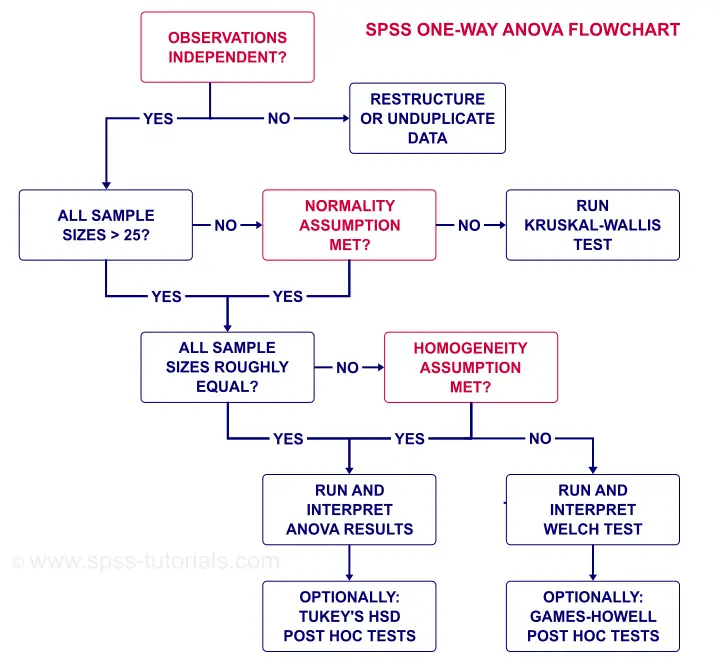
Note that depression.sav contains 4 medicine samples of n = 25 independent observations. It therefore meets all ANOVA assumptions.
SPSS ANOVA Dialogs
We'll run our ANOVA from
as shown below.
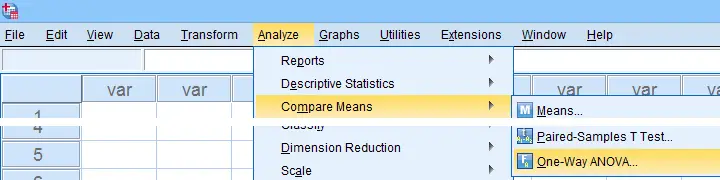
Next, let's fill out the dialogs as shown below.
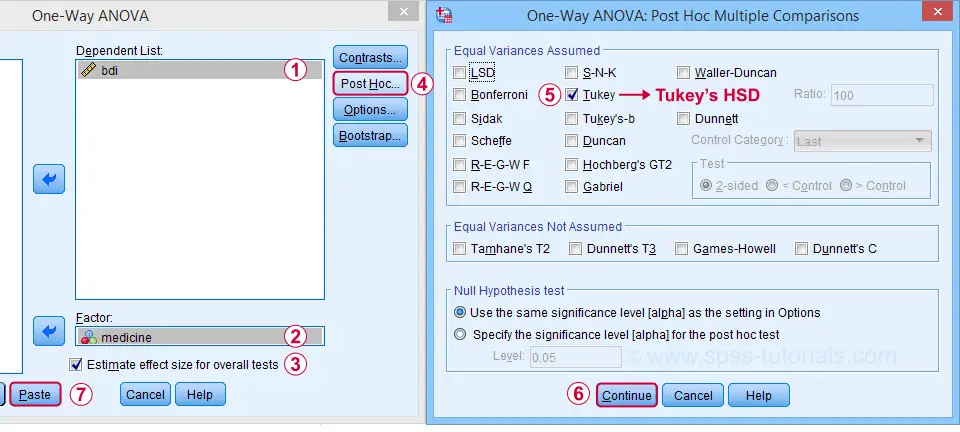
Estimate effect size(...) is only available for SPSS version 27 or higher. If you're on an older version, you can get it from
(“ANOVA table” under “Options”).
Tukey's HSD (“honestly significant difference”) is the most common post hoc test for ANOVA. It is listed under “equal variances assumed”, which refers to the homogeneity assumption. However, this is not needed for our data because our sample sizes are all equal.
Completing these steps results in the syntax below.
ONEWAY bdi BY medicine
/ES=OVERALL
/MISSING ANALYSIS
/CRITERIA=CILEVEL(0.95)
/POSTHOC=TUKEY ALPHA(0.05).
SPSS ANOVA Output
First off, the ANOVA table shown below addresses the null hypothesis that all population means are equal. The significance level indicates that p < .001 so we reject this null hypothesis. The figure below illustrates how this result should be reported.
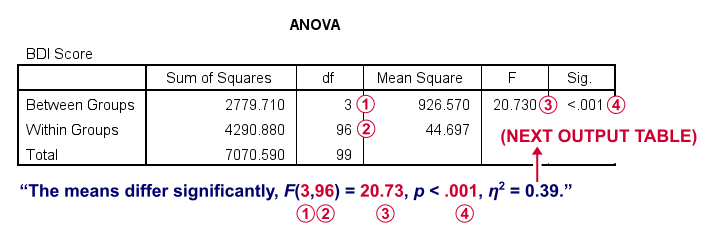
What's absent from this table, is eta squared, denoted as η2. In SPSS 27 and higher, we find this in the next output table shown below.
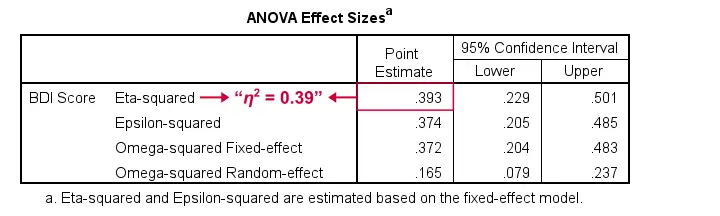
Eta-squared is an effect size measure: it is a single, standardized number that expresses how different several sample means are (that is, how far they lie apart). Generally accepted rules of thumb for eta-squared are that
- η2 = 0.01 indicates a small effect;
- η2 = 0.06 indicates a medium effect;
- η2 = 0.14 indicates a large effect.
For our example, η2 = 0.39 is a huge effect: our 4 medicines resulted in dramatically different mean depression scores.
This may seem to complete our analysis but there's one thing we don't know yet: precisely which mean differs from which mean? This final question is answered by our post hoc tests that we'll discuss next.
SPSS ANOVA - Post Hoc Tests Output
The table below shows if the difference between each pair of means is statistically significant. It also includes 95% confidence intervals for these differences.
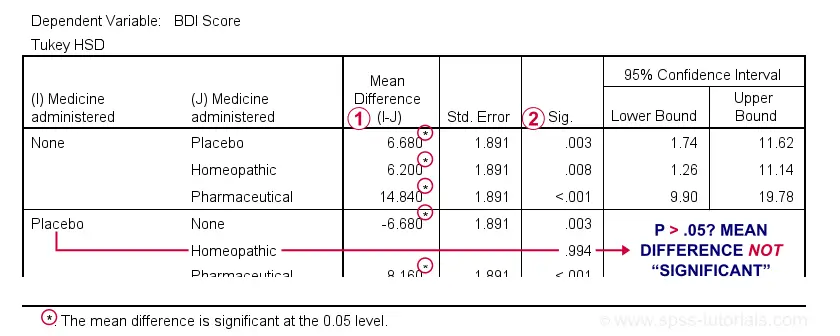
Mean differences that are “significant” at our chosen α = .05 are flagged. Note that each mean differs from each other mean except for Placebo versus Homeopathic.
If we take a good look at the exact 2-tailed p-values, we see that they're all < .01 except for the aforementioned comparison.
Given this last finding, I suggest rerunning our post hoc tests at α = .01 for reconfirming these findings. The syntax below does just that.
ONEWAY bdi BY medicine
/ES=OVERALL
/MISSING ANALYSIS
/CRITERIA=CILEVEL(0.95)
/POSTHOC=TUKEY ALPHA(0.01).
APA Style Reporting Post Hoc Tests
The table below shows how to report post hoc tests in APA style.
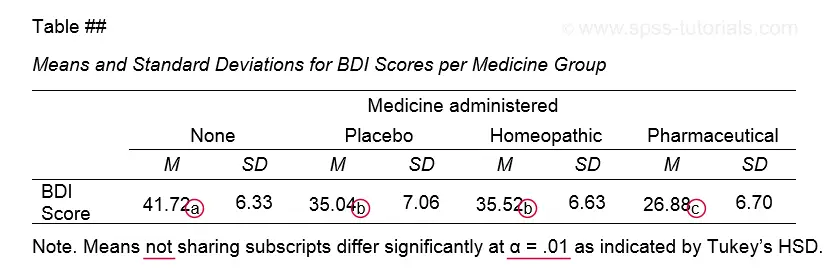
This table itself was created with a MEANS command like we used for Descriptive Statistics for Subgroups. The subscripts are based on the Homogeneous Subsets table in our ANOVA output.
Note that we chose α = .01 instead of the usual α = .05. This is simply more informative for our example analysis because all of our p-values < .05 are also < .01.
This APA table also seems available from
but I don't recommend this: the p-values from Custom Tables seem to be based on Bonferroni adjusted independent samples t-tests instead of Tukey's HSD. The general opinion on this is that this procedure is overly conservative.
Final Notes
Right, so a common routine for ANOVA with post hoc tests is
- run a basic ANOVA to see if the population means are all equal. This is often referred to as the omnibus test (omnibus is Latin, meaning something like “about all things”);
- only if we reject this overall null hypothesis, then find out precisely which pairs of means differ with post hoc tests (post hoc is Latin for “after that”).
Running post hoc tests when the omnibus test is not statistically significant is generally frowned upon. Some scenarios could perhaps justify doing so but let's leave that discussion for another day.
Right, so that should do. I hope you found this tutorial helpful. If you've any questions or remarks, please throw me a comment below. Other than that...
thanks for reading!
SPSS One-Way ANOVA Tutorial
For reading up on some basics, see ANOVA - What Is It?
- One-Way ANOVA - Null Hypothesis
- ANOVA Assumptions
- SPSS ANOVA Flowchart
- SPSS One-Way ANOVA Dialog
- SPSS ANOVA Output
- ANOVA - APA Reporting Guidelines
ANOVA Example - Effect of Fertilizers on Plants
A farmer wants to know which fertilizer is best for his parsley plants. So he tries different fertilizers on different plants and weighs these plants after 6 weeks. The data -partly shown below- are in parsley.sav.
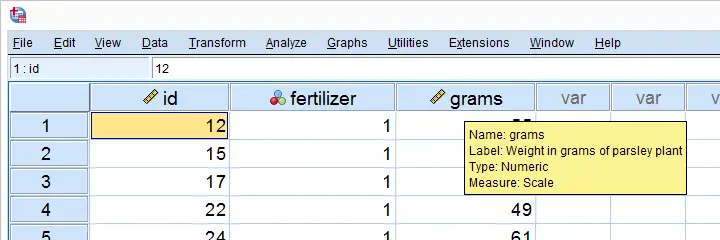
Quick Data Check - Split Histograms
After opening our data in SPSS, let's first see what they basically look like. A quick way for doing so is inspecting a histogram of weights for each fertilizer separately. The screenshot below guides you through.
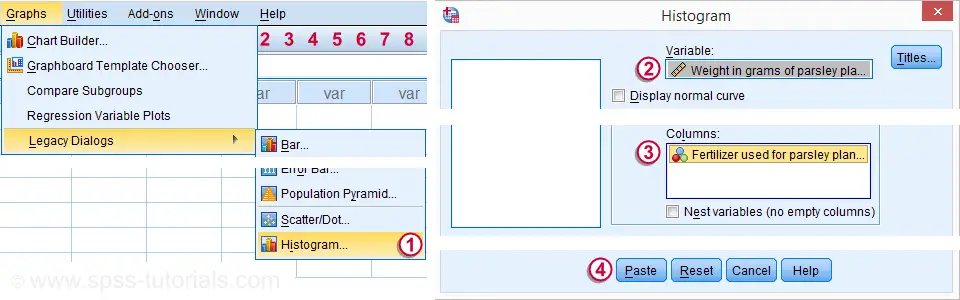
After following these steps, clicking syntax below. Let's run it.
results in theGRAPH
/HISTOGRAM=grams
/PANEL COLVAR=fertilizer COLOP=CROSS.
Result
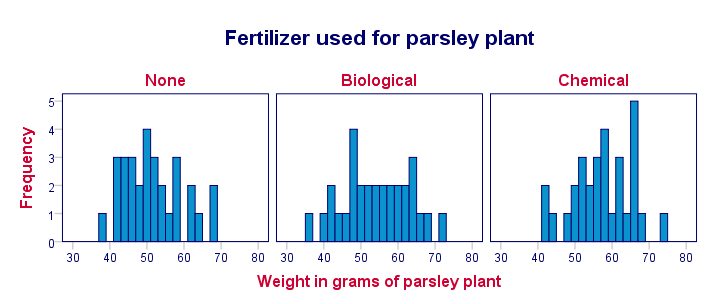
Importantly, these distributions look plausible and we don't see any outliers: our data seem correct to begin with -not always the case with real-world data!
Conclusion: the vast majority of weights are between some 40 and 65 grams and they seem reasonably normally distributed.
Inspecting Sample Sizes and Means
Precisely how did the fertilizers affect the plants? Let's compare some descriptive statistics for fertilizers separately. The quickest way is using MEANS which we could paste from
but just typing the syntax may be just as easy.
means grams by fertilizer
/cells count mean stddev.
Result
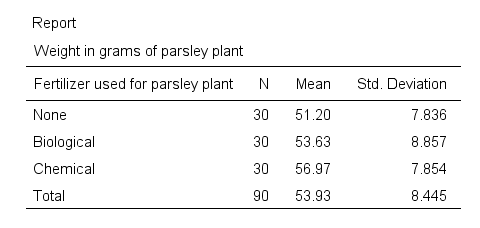
- We have sample sizes of n = 30 for each fertilizer.
- Second, the chemical fertilizer resulted in the highest mean weight of almost 57 grams. “None” performed worst at some 51 grams while “Biological” is in between.
- “Biological” has a slightly higher standard deviation than the other conditions but the difference is pretty small.
Now, this table tells us a lot about our samples of plants. But what do our sample means say about the population means? Can we say anything about the effects of fertilizers on all (future) plants? We'll try to do so by refuting the statement that all fertilizers perform equally: our null hypothesis.
One-Way ANOVA - Null Hypothesis
The null hypothesis for ANOVA is that
all population means are equal.
If this is true, then our sample means will probably differ a bit anyway. However, very different sample means contradict the hypothesis that the population means are equal. In this case, we may conclude that this null hypothesis probably wasn't true after all.
ANOVA will basically tells us to what extent our null hypothesis is credible. However, it requires some assumptions regarding our data.
ANOVA Assumptions
- independent observations: each record in the data must be a distinct and independent entity.Precisely, the assumption is “independent and identically distributed variables” but a thorough explanation is way beyond the scope of this tutorial.
- normality: the dependent variable is normally distributed in the population. Normality is not needed for reasonable sample sizes, say each n ≥ 25.
- homogeneity: the variance of the dependent variable must be equal in each subpopulation. Homogeneity is only needed for (sharply) unequal sample sizes. In this case, Levene's test can be used to see if homogeneity is met.
So how to check if we meet these assumptions? And what to do if we violate them? The simple flowchart below guides us through.
SPSS ANOVA Flowchart
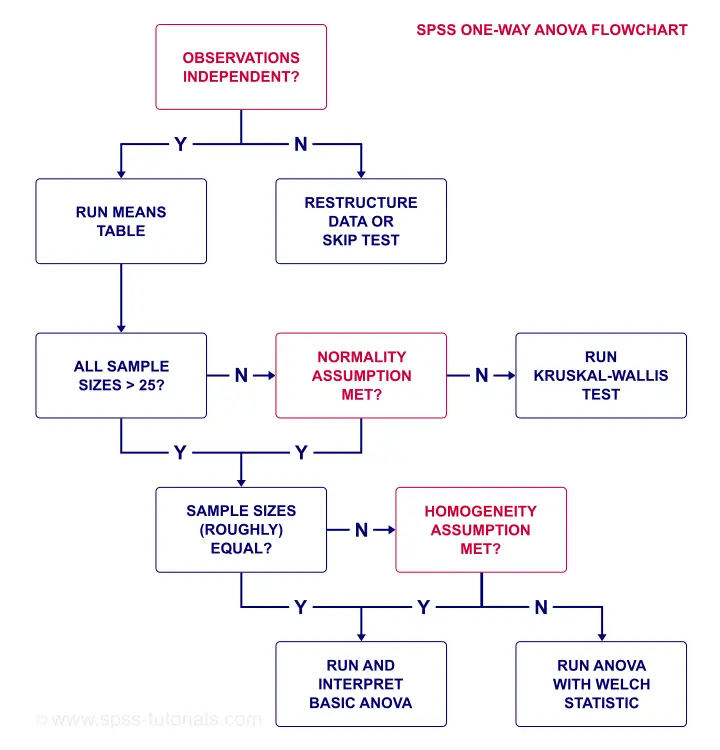
So what about our data?
- Our plants seem to be independent observations: each has a different id value (first variable).
- Our means table shows that each n ≥ 25 so we don't need to meet normality.
- Since our sample sizes are equal, we don't need the homogeneity assumption either.
So why do we inspect our sample sizes based on a means table? Why didn't we just look at the frequency distribution for fertilizer? Well, our ANOVA uses only cases without missing values on our dependent variable. And our means table shows precisely those.
A second reason is that we need to report the means and standard deviations per group. And the means table gives us precisely the statistics we want in the order we want them.
SPSS One-Way ANOVA Dialog
We'll now run a basic ANOVA from the menu. The screenshot below guides you through.
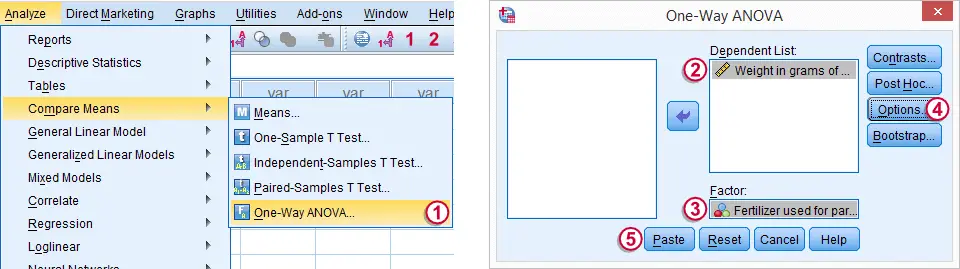
The syntax below.
button creates theOne-Way ANOVA Syntax
ONEWAY grams BY fertilizer
/MISSING ANALYSIS.
SPSS One-Way ANOVA Output
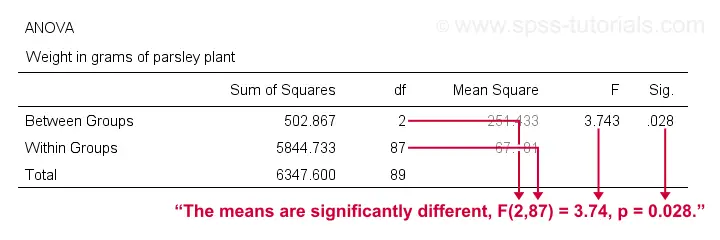
A general rule of thumb is that we
reject the null hypothesis if “Sig.” or p < 0.05
which is the case here. So we reject the null hypothesis that all population means are equal.
Conclusion: different fertilizers perform differently. The differences between our mean weights -ranging from 51 to 57 grams- are statistically significant.
ANOVA - APA Reporting Guidelines
First and foremost, we'll report our means table. Regarding the significance test, the APA suggests we report
- the F value;
- df1, the numerator degrees of freedom;
- df2, the denominator degrees of freedom;
- the p value
like so: “our three fertilizer conditions resulted in different mean weights for the parsley plants, F(2,87) = 3.7, p = .028.”
One-Way ANOVA - Next Steps
For this example, there's 2 more things we could take a look at:
- Post hoc tests: our ANOVA results tell us that not all population means are equal. But precisely which mean differs from which other mean? This is answered by running post hoc tests. For an outstanding tutorial, consult SPSS - One Way ANOVA with Post Hoc Tests Example.
- Effect size: we concluded that fertilizers affect mean weights but how strong is this effect? A common effect size measure for ANOVA is partial eta squared. Sadly, effect size is absent from the One-Way dialog.
Oddly, MEANS does include eta-squared but lacks other essential options such as Levene’s test. For complete output, you need to run your ANOVA twice from 2 different commands. This really is a major stupidity in SPSS. There. I said it.
ANOVA with Eta-Squared from MEANS
means grams by fertilizer
/statistics anova.
Result
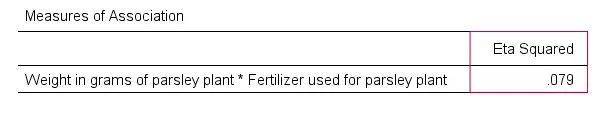
Right, so that's about the most basic SPSS ANOVA tutorial I could come up with. I hope you found it helpful. Let me know what you think by throwing me a comment below.
Thanks for reading!
ANOVA – Super Simple Introduction
- ANOVA - Null Hypothesis
- Test Statistic - F
- Assumptions for ANOVA
- Effect Size - (Partial) Eta Squared
- ANOVA - Post Hoc Tests
ANOVA -short for “analysis of variance”- is a statistical technique
for testing if 3(+) population means are all equal.
The two simplest scenarios are
- one-way ANOVA for comparing 3(+) groups on 1 variable: do all children from school A, B and C have equal mean IQ scores? For 2 groups, one-way ANOVA is identical to an independent samples t-test.
- repeated measures ANOVA for comparing 3(+) variables in 1 group: is the mean rating for beer A, B and C equal for all people?For 2 variables, repeated measures ANOVA is identical to a paired samples t-test.
The figure below visualizes the basic question for one-way ANOVA.
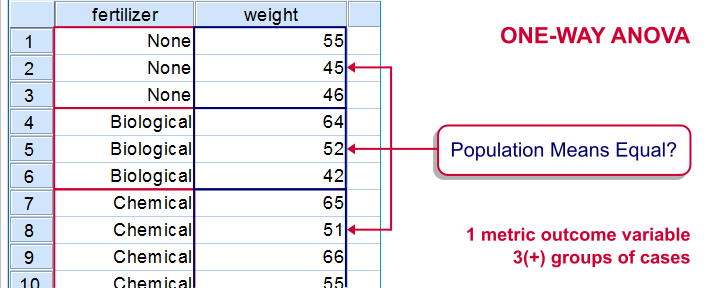
Simple Example - One-Way ANOVA
A scientist wants to know if all children from schools A, B and C have equal mean IQ scores. Each school has 1,000 children. It takes too much time and money to test all 3,000 children. So a simple random sample of n = 10 children from each school is tested.
Part of these data -available from this Googlesheet are shown below.
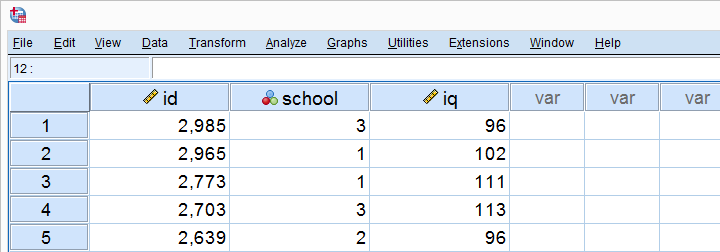
Descriptives Table
Right, so our data contain 3 samples of 10 children each with their IQ scores. Running a simple descriptives table immediately tells us the mean IQ scores for these samples. The result is shown below.
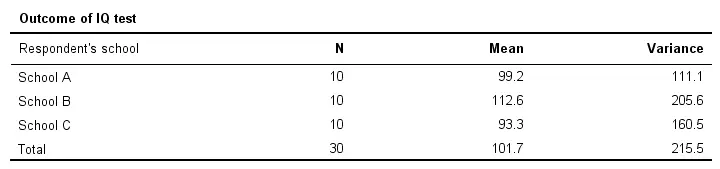
For making things clearer, let's visualize the mean IQ scores per school in a simple bar chart.
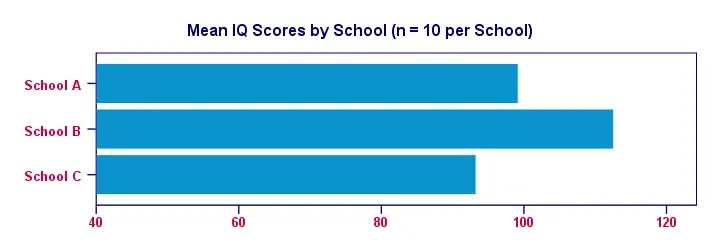
Clearly, our sample from school B has the highest mean IQ - roughly 113 points. The lowest mean IQ -some 93 points- is seen for school C.
Now, here's the problem: our mean IQ scores are only based on tiny samples of 10 children per school. So couldn't it be that
all 1,000 children per school have the same mean IQ?
Perhaps we just happened to sample the smartest children from school B and the dumbest children from school C?“Dumbest” isn't really appropriate here: these children may have terrific talents that -unfortunately for them- aren't measured by the test adminstered. However, a discussion of the usefulness of IQ tests is beyond the scope of this tutorial. Is that realistic? We'll try and show that this statement -our null hypothesis- is not credible given our data.
ANOVA - Null Hypothesis
The null hypothesis for (any) ANOVA is that all population means are exactly equal. If this holds, then our sample means will probably differ a bit. After all, samples always differ a bit from the populations they represent. However, the sample means probably shouldn't differ too much. Such an outcome would be unlikely under our null hypothesis of equal population means. So if we do find this, we'll probably no longer believe that our population means were really equal.
ANOVA - Sums of Squares Between
So precisely how different are our 3 sample means? How far do these numbers lie apart? A number that tells us just that is the variance. So we'll basically compute the variance among our 3 sample means.
As you may (or may not) understand from the ANOVA formulas, this starts with the sum of the squared deviations between the 3 sample means and the overall mean. The outcome is known as the “sums of squares between” or SSbetween. So
sums of squares between expresses
the total amount of dispersion among the sample means.
Everything else equal, larger SSbetween indicates that the sample means differ more. And the more different our sample means, the more likely that our population means differ as well.
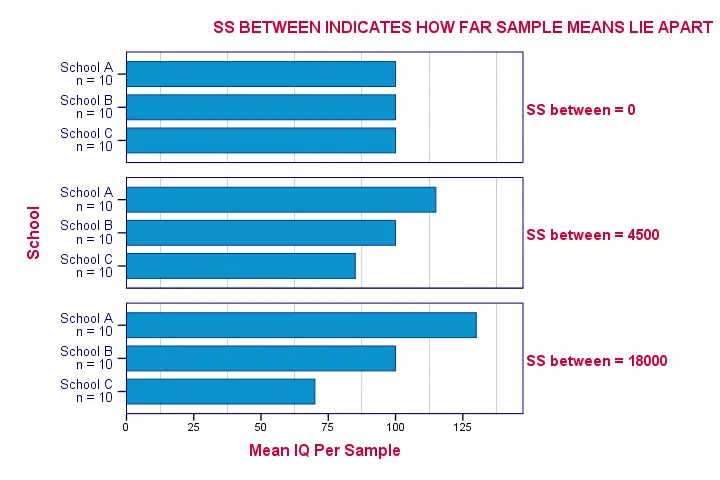
Degrees of Freedom and Mean Squares Between
When calculating a “normal” variance, we divide our sums of squares by its degrees of freedom (df). When comparing k means, the degrees of freedom (df) is (k - 1).
Dividing SSbetween by (k - 1) results in mean squares between: MSbetween. In short,
mean squares between
is basically the variance among sample means.
MSbetween thus indicates how far our sample means differ (or lie apart). The larger this variance between means, the more likely that our population means differ as well.
ANOVA - Sums of Squares Within
If our population means are really equal, then what difference between sample means -MSbetween- can we reasonably expect? Well, this depends on the variance within subpopulations. The figure below illustrates this for 3 scenarios.
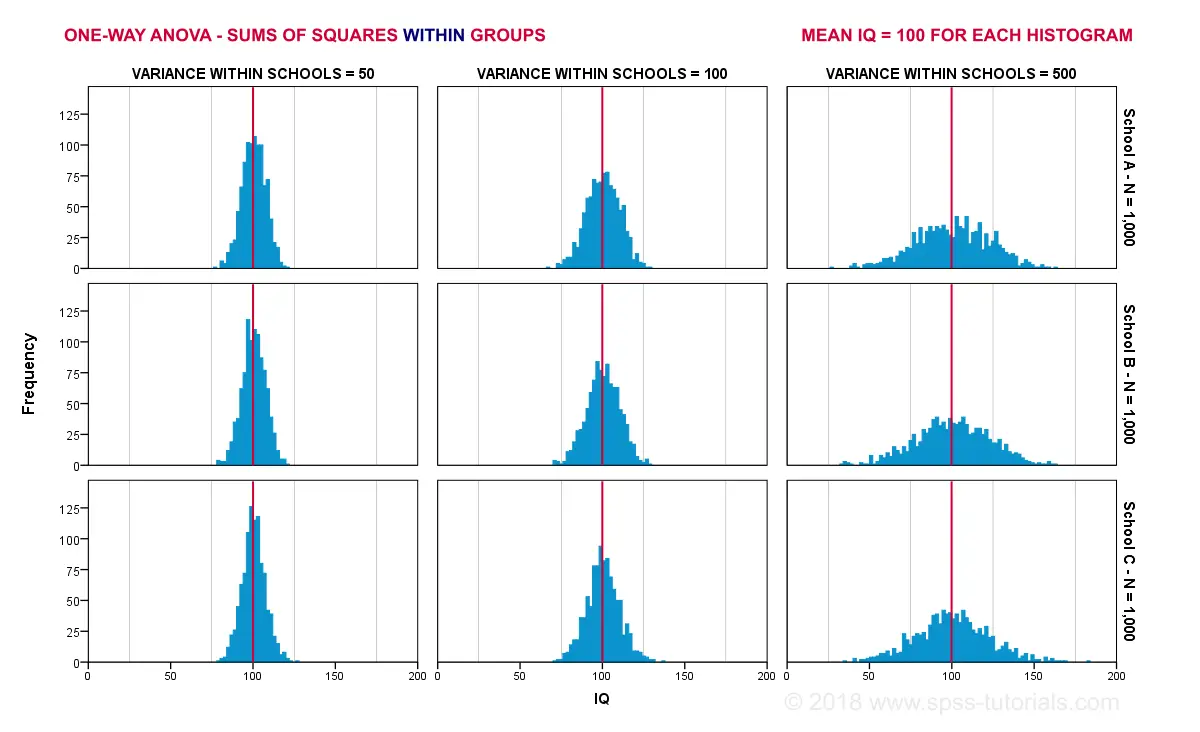
The 3 leftmost histograms show population distributions for IQ in schools A, B and C. Their narrowness indicates a small variance within each school. If we'd sample n = 10 students from each school,
should we expect very different sample means?
Probably not. Why? Well, due to the small variance within each school, the sample means will be close to the (equal) population means. These narrow histograms don't leave a lot of room for their sample means to fluctuate and -hence- differ.
The 3 rightmost histograms show the opposite scenario: the histograms are wide, indicating a large variance within each school. If we'd sample n = 10 students from each school, the means in these samples may easily differ quite a lot. In short,
larger variances within schools probably result in a
larger variance between sample means per school.
We basically estimate the within-groups population variances from the within-groups sample variances. Makes sense, right? The exact calculations are in the ANOVA formulas and this Googlesheet. In short:
- sums of squares within (SSwithin) indicates the total amount of dispersion within groups;
- degrees of freedom within (DFwithin) is (n - k) for n observations and k groups and
- mean squares within (MSwithin) -basically the variance within groups- is SSwithin / DFwithin.
ANOVA Test Statistic - F
So how likely are the population means to be equal? This depends on 3 pieces of information from our samples:
- the variance between sample means (MSbetween);
- the variance within our samples (MSwithin) and
- the sample sizes.
We basically combine all this information into a single number: our test statistic F. The diagram below shows how each piece of evidence impacts F.
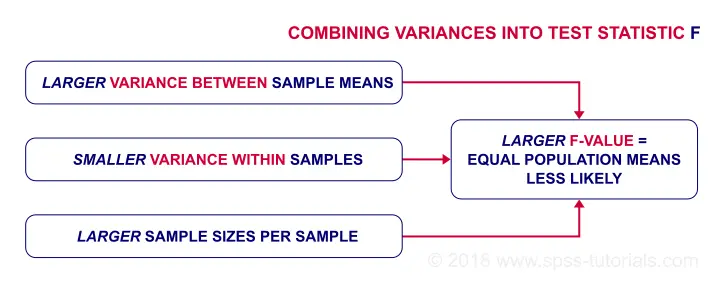
Now, F itself is not interesting at all. However, we can obtain the statistical significance from F if it follows an F-distribution. It will do just that if 3 assumptions are met.
ANOVA - Assumptions
The assumptions for ANOVA are
- independent observations;
- normality: the outcome variable must follow a normal distribution in each subpopulation. Normality is really only needed for small sample sizes, say n < 20 per group.
- homogeneity: the variances within all subpopulations must be equal. Homogeneity is only needed if sample sizes are very unequal. In this case, Levene's test indicates if it's met.
If these assumptions hold, then F follows an F-distribution with DFbetween and DFwithin degrees of freedom. In our example -3 groups of n = 10 each- that'll be F(2,27).
ANOVA - Statistical Significance
In our example, F(2,27) = 6.15. This huge F-value is strong evidence that our null hypothesis -all schools having equal mean IQ scores- is not true. If all assumptions are met, F follows the F-distribution shown below.
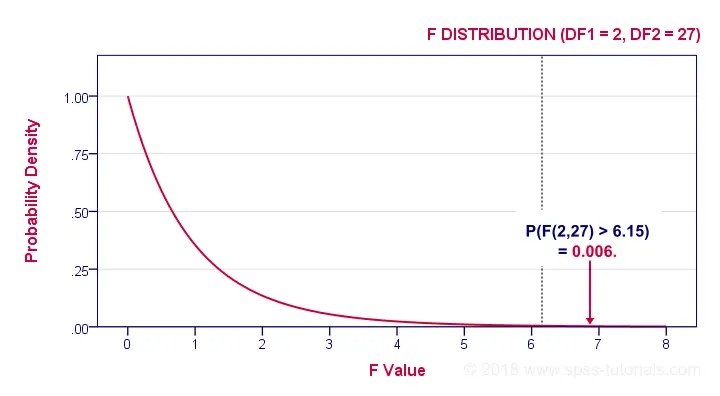
Given this distribution, we can look up that the statistical significance. We usually report: F(2,27) = 6.15, p = 0.006. If our schools have equal mean IQ's, there's only a 0.006 chance of finding our sample mean differences or larger ones. We usually say something is “statistically significant” if p < 0.05. Conclusion: our population means are very unlikely to be equal. The figure below shows how SPSS presents the output for this example.
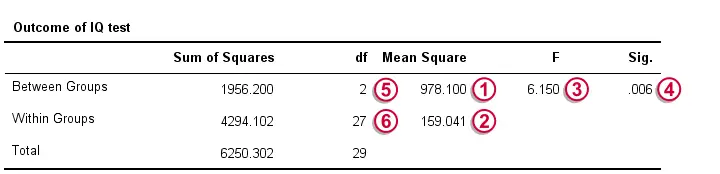
Effect Size - (Partial) Eta Squared
So far, our conclusion is that the population means are not all exactly equal. Now, “not equal” doesn't say much. What I'd like to know is
exactly how different are the means?
A number that estimates just that is the effect size. An effect size measure for ANOVA is partial eta squared, written as η2.η is the Greek letter “eta”, pronounced as a somewhat prolonged “e”. For a one-way ANOVA, partial eta-squared is equal to simply eta-squared.
Technically,
(partial) eta-squared is the
proportion of variance accounted for by a factor.
Some rules of thumb are that
- η2 > 0.01 indicates a small effect;
- η2 > 0.06 indicates a medium effect;
- η2 > 0.14 indicates a large effect.
The exact calculation of eta-squared is shown in the formulas section. For now, suffice to say that η2 = 0.31 for our example. This huge -huge- effect size explains why our F-test is statistically significant despite our very tiny sample sizes of n = 10 per school.
Post Hoc Tests - Tukey's HSD
So far, we concluded from our F-test that our population means are very unlikely to be (all) equal. The effect size, η2, told us that the difference is large. An unanswered question, though, is precisely which means are different? Different patterns of sample means may all result in the exact same F-value. The figure below illustrates this point with some possible scenarios.
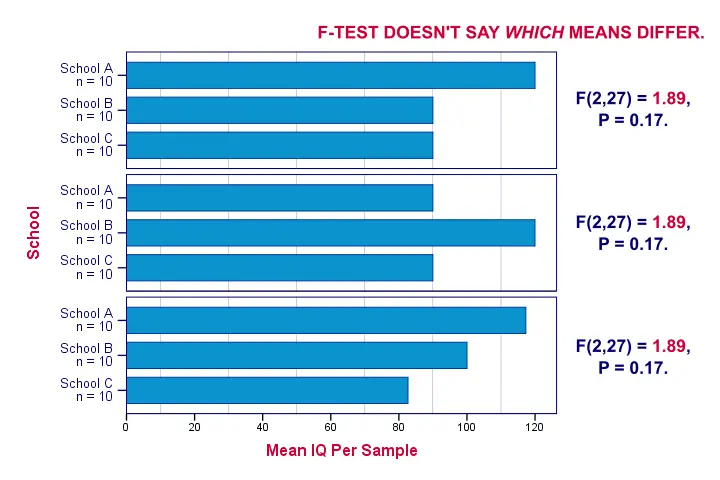
One approach would be running independent samples t-tests on all possible pairs of means. For 3 means, that'll be A-B, A-C and B-C. However, as the number of means we compare grows, the number of all possible pairs rapidly increases.Precisely, k means result in 0.5 * k * (k - 1) distinct pairs. Like so, 3 means have 3 distinct pairs, 4 means have 6 distinct pairs and 5 means have 10 distinct pairs. And
each t-test has its own chance of drawing a wrong conclusion.
So the more t-tests we run, the bigger the risk of drawing at least one wrong conclusion.
The most common solution to this problem is using Tukey's HSD (short for “Honestly Significant Difference”) procedure. You could think of it as running all possible t-tests for which the results have been corrected with some sort of Bonferroni correction but less conservative. The figure below shows some output from Tukey's HSD in SPSS.
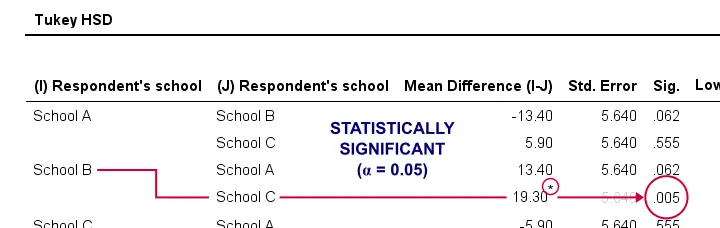
Tukey's HSD is known as a post hoc test. “Post hoc” is Latin and literally means “after that”. This is because they are run only after the main F-test has indicated that not all means are equal. I don't entirely agree with this convention because
- post hoc tests may not indicate differences while the main F-test does;
- post hoc tests may indicate differences while the main F-test does not.
Say I'm comparing 5 means: A, B, C and D are equal but E is much larger than the others. In this case, the large difference between E and the other means will be strongly diluted when testing if all means are equal. So in this case
an overall F-test may not indicate any differences
while post hoc tests will.
Last but not least, there's many other post hoc tests as well. Some require the homogeneity assumption and others don't. The figure below shows some examples.
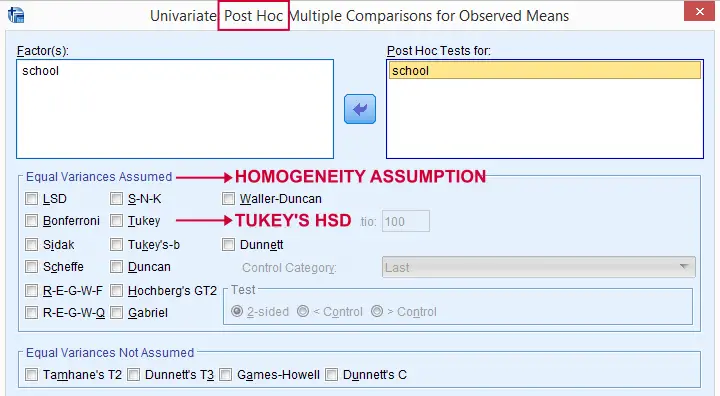
ANOVA - Basic Formulas
For the sake of completeness, we'll list the main formulas used for the one-way ANOVA in our example. You can see them in action in this Googlesheet.
We'll start off with the between-groups variance:
$$SS_{between} = \Sigma\;n_j\;(\overline{X}_j - \overline{X})^2$$
where
- \(\overline{X}_j\) denotes a group mean;
- \(\overline{X}\) is the overall mean;
- \(n_j\) is the sample size per group.
For our example, this results in
$$SS_{between} = 10\;(99.2 - 101.7)^2 + 10\;(112.6 - 101.7)^2 + 10\;(93.3 - 101.7)^2 = 1956.2 $$
Next, for \(m\) groups,
$$df_{between} = m - 1$$
so \(df_{between}\) = 3 - 1 = 2 for our example data.
$$MS_{between} = \frac{SS_{between}}{df_{between}}$$
For our example, that'll be
$$\frac{1956.2}{2} = 978.1$$
We now turn to the within-groups variance. First off,
$$SS_{within} = \Sigma\;(X_i - \overline{X}_j)^2$$
where
- \(\overline{X}_j\) denotes a group mean;
- \(X_i\) denotes an individual observation (“data point”).
For our example, this'll be
$$SS_{within} = (90 - 99.2)^2 + (87 - 99.2)^2 + ... + (96 - 93.3)^2 = 4294.1$$
for \(n\) independent observations and \(m\) groups,
$$df_{within} = n - m$$
So for our example that'll be = 30 - 3 = 27.
$$MS_{within} = \frac{SS_{within}}{df_{within}}$$
For our example, this results in
$$\frac{4294.1}{27} = 159$$
We're now ready to calculate the F-statistic:
$$F = \frac{MS_{between}}{MS_{within}}$$
which results in
$$\frac{978.1}{159} = 6.15$$
Finally,
$$P = P(F(2,27) > 6.15) = 0.0063$$
Optionally, the effect size η2 is calculated as
$$Effect\;\;size\;\;\eta^2 = \frac{SS_{between}}{SS_{between} + SS_{within}}$$
For our example, that'll be
$$\frac{1956.2}{1956.2 + 4294.1} = 0.31$$
Thanks for reading.